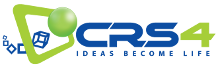
Non-intrusive load disaggregation by convolutional neural network and multilabel classification
Applied Sciences, Volume 10, page 1454 - february 2020
Télécharger la publication :
Non-intrusive load monitoring (NILM) is the main method used to monitor the energy footprint of a residential building and disaggregate total electrical usage into appliance-related signals. The most common disaggregation algorithms are based on the Hidden Markov Model, while solutions based on deep neural networks have recently caught the attention of researchers. In this work we address the problem through the recognition of the state of activation of the appliances using a fully convolutional deep neural network, borrowing some techniques used in the semantic segmentation of images and multilabel classification. This approach has allowed obtaining high performances not only in the recognition of the activation state of the domestic appliances but also in the estimation of their consumptions, improving the state of the art for a reference dataset.
Références BibTex
@Article{MMM20,
author = {Massidda, L. and Marrocu, M. and Manca, S.},
title = {Non-intrusive load disaggregation by convolutional neural network and multilabel classification},
journal = {Applied Sciences},
volume = {10},
pages = {1454},
month = {february},
year = {2020},
publisher = {MDPI},
keywords = {energy disaggregation, non-intrusive load monitoring, convolutional neural network, deep learning},
doi = {10.3390/app10041454 },
url = {https://publications.crs4.it/pubdocs/2020/MMM20},
}
Autres publications dans la base