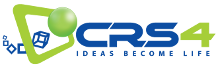
QQS: a method to reconstruct multiple time series of daily cumulated precipitation.
Rapport de recherche , CRS4 - june 2014
Long Abstract. The most important element with which fed a surface hydrological model are the precipitation data. Time series of measured data within the catchment of interest must be representative of the time and spatial distribution of the precipitation field and must have no missing data for the period under study. It happens very seldom that time series are instead incomplete or, when operating with data coming from a meteorological or a climate model, are strongly biased and moreover don't have the necessary resolution to describe the spatial distribution of the precipitation field within the catchment. For these reasons, when needed, it is important to be able to reconstruct, completely or partially, time series of precipitation with time and spatial statistics that match the “correct” ones, estimated during periods when data are available.
The simplest approach is that of generating a completely synthetic time series using a stochastic process able to reproduce the main important statistical parameters that for precipitation are typically wet/dry duration periods and related probability of transition and the probability distribution function with particular care devoted to the tail. This approach has the drawback that the time sequence of the reconstructed precipitation events is completely random and any kind of correlation with other physical parameters (for example temperature, wind and relative humidity) is lost.
To avoid such a problem a realistic “triggering” signal for the precipitation events can be obtained using data from meteorological reanalysis (for the past) or from meteorological or climatic numerical models (for the future).
Within the “Bilancio Idrologico” project, that has the main objective of reconstructing the past hydrological balance (years from 1922 to 2010) in condition of naturality and for the entire Sardinia Island, and in view of applying the same procedure for studies of future climate scenario, we developed a methodology of precipitation reconstruction at daily scale using precipitation data of the ECMWF ERA-interim reanalysis project. That data although accurate describing the temporal behavior of the precipitation events are seasonally biased and the seasonal probability distribution function (pdf) must be necessarily corrected.
To this achievement, despite many limitations, the daily translation method has been shown to be skillful in many hydrologic studies (see for example Wood et al., 2004; Maurer and Hidalgo, 2008). In this method the cumulative distribution function of analysis data is compared with that of the corresponding measured data, using the so called quantile-quantile scatter plot (QQ correction method, from now on). The value needed to exactly map the quantile of the measured data to the corresponding quantile of the analyzed ones is added to these last data. This assure that, at least in the calibration period when observations are available, the moments of the probability distribution function of the corrected analyzed/modelled data are coincident with that of measured data.
This procedure can be applied separately to each raingage station. Due to the low spatial resolution of the analysis field (about 70kmx70km), being each time series “triggered” with essentially the same signal for relatively smaller areas, resulting spatial correlation between time series will be unrealistically high. To avoid this problem we coupled to the QQ correction method an algorithm able to rebuild the correct spatial correlation. The method, we devised, derives from the Shackle-shuffling methodology and it consists in shuffling for each time step the members of an ensemble of equiprobable realizations obtained assigning some uncertainty to the QQ correction. The degree of uncertainty can be increased (decreased), reducing (increasing) the number of quantiles used when applying the QQ correction. The shuffling is made in such a way to reproduce the relative rank between real measurements at fixed time. The net effect of this re-arrangement of ensemble members is that of reproducing a realistic cross-correlation between different time series.
As far as we know the procedure, that we will call QQS (quantile-quantile and shuffling), has never been proposed before. The results obtained and discussed in this report seem to be encouraging and prove that, at least, for a limited number of stations, spatially distributed, the QQS algorithm is able to reproduce both, the main statistics parameters of the single time series, and the correct spatial correlation between them.
Références BibTex
@TechReport{Mar14,
author = {Marrocu, M.},
title = {QQS: a method to reconstruct multiple time series of daily cumulated precipitation.},
institution = {CRS4},
month = {june},
year = {2014},
keywords = {time series, preciitation, climatological scenario},
url = {https://publications.crs4.it/pubdocs/2014/Mar14},
}
Autres publications dans la base