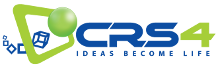
Quantile Regression Post-Processing of Weather Forecast for Short-Term Solar Power Probabilistic Forecasting
Energies, Volume 11, Number 7, page 1763 - july 2018
Télécharger la publication :
The inclusion of photo-voltaic generation in the distribution grid poses technical difficulties related to the variability of the solar source and determines the need for Probabilistic Forecasting procedures (PF). This work describes a new approach for PF based on quantile regression using the Gradient-Boosted Regression Trees (GBRT) method fed by numerical weather forecasts of the European Centre for Medium Range Weather Forecast (ECMWF) Integrated Forecasting System (IFS) and Ensemble Prediction System (EPS). The proposed methodology is compared with the forecasts obtained with Quantile Regression using only IFS forecasts (QR), with the uncalibrated EPS forecasts and with the EPS forecasts calibrated with a Variance Deficit (VD) procedure. The proposed methodology produces forecasts with a temporal resolution equal to or better than the meteorological forecast (1 h for the IFS and 3 h for EPS) and, in the case examined, is able to provide higher performances than those obtained with the other methods over a forecast horizon of up to 72 h.
Références BibTex
@Article{MM18a,
author = {Massidda, L. and Marrocu, M.},
title = {Quantile Regression Post-Processing of Weather Forecast for Short-Term Solar Power Probabilistic Forecasting},
journal = {Energies},
number = {7},
volume = {11},
pages = {1763},
month = {july},
year = {2018},
publisher = {MDPI},
keywords = {PV power forecasting, ensemble forecasting, probabilistic forecasting},
doi = {10.3390/en11071763},
url = {https://publications.crs4.it/pubdocs/2018/MM18a},
}
Autres publications dans la base