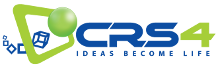
Deep Learning Approach for Detecting Regional Wall Motion Abnormality from Echocardiographic Images
Federico Caredda,
Jose Francisco Saenz Cogollo,
Maurizio Agelli
Unpublished - december 2020
Télécharger la publication :
Detecting heart motion abnormalities on echocardiographic images is crucial for an early detection of myocardial infarction in many emergency situations. However, the interpretation of the ultrasound images can be very challenging even for expert cardiologists. In this study, we propose a fully deep learning approach for detecting the presence of regional wall motion abnormalities on ultrasound sequences acquired from any of the three standard apical views used in echocardiography. We implement a two stage approach where the image sequences are first segmented by an encode-decoder network in order to isolate the left ventricular wall, and successively analyzed by a combined convolutional-recurrent network that classifies the motion of the heart muscle. The results obtained on a dataset constructed from examinations of more than 120 patients shows a very high accuracy and sensitivity.
Références BibTex
@Unpublished{CSA20,
author = {Caredda, F. and Saenz Cogollo, J. and Agelli, M.},
title = {Deep Learning Approach for Detecting Regional Wall Motion Abnormality from Echocardiographic Images},
month = {december},
year = {2020},
note = {This is a preliminary result of a research in progress},
keywords = {Deep learning, echocardiography, medical ultrasound},
url = {https://publications.crs4.it/pubdocs/2020/CSA20},
}
Autres publications dans la base