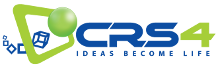
Non-intrusive load disaggregation via a fully convolutional neural network: improving the accuracy on unseen household
2020 2nd IEEE International Conference on Industrial Electronics for Sustainable Energy Systems (IESES), Volume 1, page 317-322 - september 2020
The application of load disaggregation techniques based on neural networks is often limited to users included in the training dataset. A methodology based on techniques typical of the semantic segmentation of images has been proposed for this task, which allows to obtain a high accuracy and good generalization. We introduce here a novel data augmentation technique for improving forecasts for unmonitored houses that does not require any interaction with the user, nor further measurements of consumption of household appliances.
Références BibTex
@InProceedings{MMM20a,
author = {Massidda, L. and Marrocu, M. and Manca, S.},
title = {Non-intrusive load disaggregation via a fully convolutional neural network: improving the accuracy on unseen household},
booktitle = {2020 2nd IEEE International Conference on Industrial Electronics for Sustainable Energy Systems (IESES)},
volume = {1},
pages = {317-322},
month = {september},
year = {2020},
editor = {IEEE},
publisher = {IEEE},
organization = {IEEE},
keywords = {energy disaggregation, non-intrusive load monitoring, convolutional neural network, deep learning},
doi = {10.1109/IESES45645.2020.9210661},
url = {https://publications.crs4.it/pubdocs/2020/MMM20a},
}
Autres publications dans la base