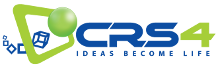
AtlantaNet: Inferring the 3D Indoor Layout from a Single 360 Image beyond the Manhattan World Assumption
Proc. ECCV, page 432--448 - august 2020
Télécharger la publication :
We introduce a novel end-to-end approach to predict a 3D room layout from a single panoramic image. Compared to recent state-of-the-art works, our method is not limited to Manhattan World environments, and can reconstruct rooms bounded by vertical walls that do not form right angles or are curved - i.e., Atlanta World models. In our approach, we project the original gravity-aligned panoramic image on two horizontal planes, one above and one below the camera. This representation encodes all the information needed to recover the Atlanta World 3D bounding surfaces of the room in the form of a 2D room footprint on the floor plan and a room height. To predict the 3D layout, we propose an encoder-decoder neural network architecture, leveraging Recurrent Neural Networks (RNNs) to capture long-range geometric patterns, and exploiting a customized training strategy based on domain-specific knowledge. The experimental results demonstrate that our method outperforms state-of-the-art solutions in prediction accuracy, in particular in cases of complex wall layouts or curved wall footprints.
Images et films
Références BibTex
@InProceedings{PAG20,
author = {Pintore, G. and Agus, M. and Gobbetti, E.},
title = {AtlantaNet: Inferring the 3D Indoor Layout from a Single 360 Image beyond the Manhattan World Assumption},
booktitle = {Proc. ECCV},
pages = {432--448},
month = {august},
year = {2020},
keywords = {3D floor plan recovery, panoramic images, 360 images, datadriven reconstruction, structured indoor reconstruction, indoor panorama, room layout estimation, holistic scene structure},
doi = {10.1145/978-3-030-58598-3_26},
url = {https://publications.crs4.it/pubdocs/2020/PAG20},
}
Autres publications dans la base