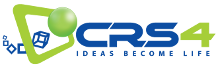
Investigating Feature Selection and Random Forests for Inter-Patient Heartbeat Classification
Jose Francisco Saenz Cogollo,
Maurizio Agelli
Algorithms, Volume 13, Number 4, page 75 - april 2020
Finding an optimal combination of features and classifier is still an open problem in the development of automatic heartbeat classification systems, especially when applications that involve resource-constrained devices are considered. In this paper, a novel study of the selection of informative features and the use of a random forest classifier while following the recommendations of the Association for the Advancement of Medical Instrumentation (AAMI) and an inter-patient division of datasets is presented. Features were selected using a filter method based on the mutual information ranking criterion on the training set. Results showed that normalized beat-to-beat (R–R) intervals and features relative to the width of the ventricular depolarization waves (QRS complex) are the most discriminative among those considered. The best results achieved on the MIT-BIH Arrhythmia Database were an overall accuracy of 96.14% and F1-scores of 97.97%, 73.06%, and 90.85% in the classification of normal beats, supraventricular ectopic beats, and ventricular ectopic beats, respectively. In comparison with other state-of-the-art approaches tested under similar constraints, this work represents one of the highest performances reported to date while relying on a very small feature vector.
Références BibTex
@Article{SA20,
author = {Saenz Cogollo, J. and Agelli, M.},
title = {Investigating Feature Selection and Random Forests for Inter-Patient Heartbeat Classification},
journal = {Algorithms},
number = {4},
volume = {13},
pages = {75},
month = {april},
year = {2020},
publisher = {MDPI},
keywords = {ECG feature selection, heartbeat classification, arrhythmia detection, random forest classifier},
doi = {10.3390/a13040075},
url = {https://publications.crs4.it/pubdocs/2020/SA20},
}
Autres publications dans la base