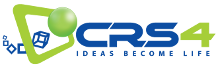
Probabilistic day-ahead prediction of PV generation. A comparative analysis of forecasting methodologies and of the factors influencing accuracy
Solar Energy, Volume 271, page 112422 - march 2024
Photovoltaic (PV) power forecasting is essential for the integration of renewable energy sources into the grid and for the optimisation of energy management systems. In this paper, we address the problem of probabilistic day-ahead forecasting of PV power generation for an operating plant with imperfect measurements and incomplete information. We compare four probabilistic forecasting methodologies: one physical irradiance- to-power method based on a model of the power plant and on weather forecasts, and four statistical methods based on quantile regression and classification techniques. We evaluate the performance of these methods in terms of deterministic and probabilistic accuracy, as well as the influence of the forecast horizon and the autoregressive component. The results show that statistical methods outperform the physical method, that conformalized quantile regression achieves the highest probabilistic accuracy, and that weather forecasts are more important than autoregressive predictors for the forecast procedure. To our knowledge, this is one of the first studies to compare different probabilistic forecasting approaches on the same case and provides information on the relative importance of the factors affecting the accuracy of the forecast.
Images et films
Références BibTex
@Article{MBM24,
author = {Massidda, L. and Bettio, F. and Marrocu, M.},
title = {Probabilistic day-ahead prediction of PV generation. A comparative analysis of forecasting methodologies and of the factors influencing accuracy},
journal = {Solar Energy},
volume = {271},
pages = {112422},
month = {march},
year = {2024},
publisher = {Elsevier Ltd},
keywords = {Probabilistic solar forecasting, Photovoltaic power, Conformalized quantile regression, Gradient boosting, Neural network classifier},
doi = {https://doi.org/10.1016/j.solener.2024.112422},
url = {https://publications.crs4.it/pubdocs/2024/MBM24},
}
Autres publications dans la base