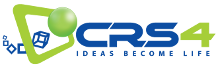
Deep Panoramic Depth Prediction and Completion for Indoor Scenes
Computational Visual Media - february 2024
Télécharger la publication :
We introduce a novel end-to-end deep learning solution for rapidly estimating a dense spherical depth map of an indoor environment. Our input is a single equirectangular image registered with a sparse depth map, as provided by a variety of common capture setups. Depth is inferred by an efficient and lightweight single-branch network, which employs a dynamic gating system to process together dense visual data and sparse geometric data. We exploit the characteristics of typical man-made environments to efficiently compress multi-resolution features and find short- and long-range relations among scene parts. Furthermore, we introduce a new augmentation strategy to make the model robust to different types of sparsity, including those generated by various structured light sensors and LiDAR setups. The experimental results demonstrate that our method provides interactive performance and outperforms state-of-the-art solutions in computational efficiency, adaptivity to variable depth sparsity patterns, and prediction accuracy for challenging indoor data, even when trained solely on synthetic data without any fine tuning.
Images et films
Références BibTex
@Article{PASVG24,
author = {Pintore, G. and Almansa, E. and Sanchez, A. and Vassena, G. and Gobbetti, E.},
title = {Deep Panoramic Depth Prediction and Completion for Indoor Scenes},
journal = {Computational Visual Media},
month = {february},
year = {2024},
keywords = {visual computing},
doi = {10.1007/s41095-023-0358-0},
url = {https://publications.crs4.it/pubdocs/2024/PASVG24},
}
Autres publications dans la base