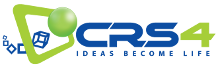
Deep scene synthesis of Atlanta-world interiors from a single omnidirectional image
IEEE Transactions on Visualization and Computer Graphics, Volume 29 - november 2023
Télécharger la publication :
We present a new data-driven approach for extracting geometric and structural information from a single spherical panorama of an interior scene, and for using this information to render the scene from novel points of view, enhancing 3D immersion in VR applications. The approach copes with the inherent ambiguities of single-image geometry estimation and novel view synthesis by focusing on the very common case of Atlanta-world interiors, bounded by horizontal floors and ceilings and vertical walls. Based on this prior, we introduce a novel end-to-end deep learning approach to jointly estimate the depth and the underlying room structure of the scene. The prior guides the design of the network and of novel domain-specific loss functions, shifting the major computational load on a training phase that exploits available large-scale synthetic panoramic imagery. An extremely lightweight network uses geometric and structural information to infer novel panoramic views from translated positions at interactive rates, from which perspective views matching head rotations are produced and upsampled to the display size. As a result, our method automatically produces new poses around the original camera at interactive rates, within a working area suitable for producing depth cues for VR applications, especially when using head-mounted displays connected to graphics servers. The extracted floor plan and 3D wall structure can also be used to support room exploration. The experimental results demonstrate that our method provides low-latency performance and improves over current state-of-the-art solutions in prediction accuracy on available commonly used indoor panoramic benchmarks.
Images et films
Références BibTex
@Article{PBAG23,
author = {Pintore, G. and Bettio, F. and Agus, M. and Gobbetti, E.},
title = {Deep scene synthesis of Atlanta-world interiors from a single omnidirectional image},
journal = {IEEE Transactions on Visualization and Computer Graphics},
volume = {29},
month = {november},
year = {2023},
note = {Proc. ISMAR.},
keywords = {visual computing},
doi = {10.1109/TVCG.2023.3320219},
url = {https://publications.crs4.it/pubdocs/2023/PBAG23},
}
Autres publications dans la base