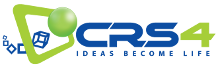
Disk-NeuralRTI: Optimized NeuralRTI Relighting through Knowledge Distillation
STAG: Smart Tools and Applications in Graphics - november 2024
Télécharger la publication :
Relightable images created from Multi-Light Image Collections (MLICs) are among the most employed models for interactive object exploration in cultural heritage (CH). In recent years, neural representations have been shown to produce higher-quality images at similar storage costs to the more classic analytical models such as Polynomial Texture Maps (PTM) or Hemispherical Harmonics (HSH). However, the Neural RTI models proposed in the literature perform the image relighting with decoder networks with a high number of parameters, making decoding slower than for classical methods. Despite recent efforts targeting model reduction and multi-resolution adaptive rendering, exploring high-resolution images, especially on high-pixel-count displays, still requires significant resources and is only achievable through progressive rendering in typical setups. In this work, we show how, by using knowledge distillation from an original (teacher) Neural RTI network, it is possible to create a more efficient RTI decoder (student network). We evaluated the performance of the network compression approach on existing RTI relighting benchmarks, including both synthetic and real datasets, and on novel acquisitions of high-resolution images. Experimental results show that we can keep the student prediction close to the teacher with up to 80% parameter reduction and almost ten times faster rendering when embedded in an online viewer.
Images et films
Références BibTex
@InProceedings{DRPGG24,
author = {Dulache, T. and Righetto, L. and Pintus, R. and Gobbetti, E. and Giachetti, A.},
title = {Disk-NeuralRTI: Optimized NeuralRTI Relighting through Knowledge Distillation},
booktitle = {STAG: Smart Tools and Applications in Graphics},
month = {november},
year = {2024},
organization = {Eurographics},
keywords = {visual computing, cultural heritage},
doi = {10.2312/stag.20241340},
url = {https://publications.crs4.it/pubdocs/2024/DRPGG24},
}
Autres publications dans la base