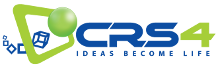
Emerging Developments in Real-Time Edge AIoT for Agricultural Image Classification
Maurizio Pintus,
Felice Colucci,
Fabio Maggio
IoT, Volume 6, Number 1 - february 2025
Advances in deep learning (DL) models and next-generation edge devices enable
real-time image classification, driving a transition from the traditional, purely cloud-centric
IoT approach to edge-based AIoT, with cloud resources reserved for long-term data storage
and in-depth analysis. This innovation is transformative for agriculture, enabling au-
tonomous monitoring, localized decision making, early emergency detection, and precise
chemical application, thereby reducing costs and minimizing environmental and health
impacts. The workflow of an edge-based AIoT system for agricultural monitoring involves
two main steps: optimal training and tuning of DL models through extensive experiments
on high-performance AI-specialized computers, followed by effective customization for
deployment on advanced edge devices. This review highlights key challenges in prac-
tical applications, including: (i) the limited availability of agricultural data, particularly
due to seasonality, addressed through public datasets and synthetic image generation;
(ii) the selection of state-of-the-art computer vision algorithms that balance high accu-
racy with compatibility for resource-constrained devices; (iii) the deployment of models
through algorithm optimization and integration of next-generation hardware accelerators
for DL inference; and (iv) recent advancements in AI models for image classification that,
while not yet fully deployable, offer promising near-term improvements in performance
and functionality.
Références BibTex
@Article{PCM25a,
author = {Pintus, M. and Colucci, F. and Maggio, F.},
title = {Emerging Developments in Real-Time Edge AIoT for Agricultural Image Classification},
journal = {IoT},
number = {1},
volume = {6},
month = {february},
year = {2025},
publisher = {MDPI},
keywords = {precision agriculture, crop continuos monitoring, image classification, edge-based AIoT, real-time deep learning, hardware accelerators, synthetic datasets, wireless communication, KANs, XNets},
doi = {10.3390/iot6010013},
url = {https://publications.crs4.it/pubdocs/2025/PCM25a},
}
Autres publications dans la base