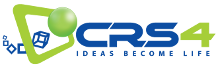
NadirFloorNet: reconstructing multi-room floorplans from a small set of registered panoramic images
2nd CVPR Workshop on Urban Scene Modeling - 2025
We introduce a novel deep-learning approach for predicting complex indoor floor plans with ceiling heights from a minimal set of registered 360-degree images of cluttered rooms. Leveraging the broad contextual information available in a single panoramic image and the availability of annotated training datasets of room layouts, a transformer-based neural network predicts a geometric representation of each room’s
architectural structure, excluding furniture and objects, and projects it on a horizontal plane (the Nadir plane) to estimate the disoccluded floor area and the ceiling heights. We then merge and process these Nadir representations on the same floor plan, using a deformable attention transformer that exploits mutual information to resolve structural occlusions and complete room reconstruction. This fully data-
driven solution achieves state-of-the-art results on synthetic and real-world datasets with a minimal number of input images.
Images et films
Références BibTex
@InProceedings{PSAG25,
author = {Pintore, G. and Shah, U. and Agus, M. and Gobbetti, E.},
title = {NadirFloorNet: reconstructing multi-room floorplans from a small set of registered panoramic images},
booktitle = {2nd CVPR Workshop on Urban Scene Modeling},
year = {2025},
publisher = {IEEE},
keywords = {visual computing, data-intensive computing},
url = {https://publications.crs4.it/pubdocs/2025/PSAG25},
}
Autres publications dans la base